“This time I won’t make any silly jokes or references”, that’s what SHE said !
In today’s article, I’m gonna try to explain to you what I’ve been doing for the last week, and any comment or suggestion would be great, even if you feel that you are not concerned, maybe you are looking at it from an angle that could help, and you can find the the full code in this link ( make sure to choose the branch : “local-search-rl” ).
Anyway, in this part of the code, you can see that there’s the initial local search function and the q-local search one, it’s good to maintain the extensibility of the code, so when I need to implement a new algorithm, all I have to do is choose between them since they do not affect the other parts.
def bso(self):i=0while(i<self.maxIterations):print("refSol is : ",self.refSolution.solution)self.tabou.append(self.refSolution)print("Iteration N° : ",i)self.searchArea()print("********************************************************")print("Search zone is :")for k in range (self.nbrBees):print(self.beeList[k].id)print(self.beeList[k].solution)print("*******************************************************")#The local searchfor j in range(self.nbrBees):self.beeList[j].reward = 0self.beeList[j].localSearch() # This is the initial local searchself.beeList[j].ql_localSearch() # This is the q-local searchprint( "Fitness of bee " + str(j) + " " + str(self.beeList[j].fitness) )self.refSolution=self.selectRefSol()i+=1
If we consider the initial algorithm of local search at the level of a bee, it does an exhaustive search, in other words it takes the solution, flips all its bits 1 by 1, and evaluates each time, which makes the complexity, if we take it as the cost of training a model, equivalent to the number of attributes of the model (for example the sonar dataset, has 60 attributes, this means we would train 60 models ) and this is only for a single bee, and for a single iteration, which makes the complexity : o(Max Iter x Nbr Bees x Nbr Local Iter x Nbr Attributes)
This is the initial local search function :
def localSearch(self):best=self.fitness#done=Falselista=[j for j, n in enumerate(self.solution) if n == 1]indice =lista[0]for itr in range(self.locIterations):while(True):pos=-1oldFitness=self.fitnessfor i in range(len(self.solution)):if ((len(lista)==1) and (indice==i)and (i < self.data.nb_attribs-1)):i+=1self.solution[i]= (self.solution[i] + 1) % 2quality = self.data.evaluate(self.solution)if (quality >best):pos = ibest=qualityself.solution[i]= (self.solution[i]+1) % 2self.fitness = oldFitnessif (pos != -1):self.solution[pos]= (self.solution[pos]+1)%2self.fitness = bestelse:breakfor i in range(len(self.solution)):oldFitness=self.fitnessif ((len(lista)==1) and (indice==i) and (i < self.data.nb_attribs-1)):i+=1self.solution[i]= (self.solution[i] + 1) % 2quality = self.data.evaluate(self.solution)if (quality<best):self.solution[i]= (self.solution[i] + 1) % 2self.fitness = oldFitness
The idea behind Q-LocalSearch is to provide a sub-set of “filtered” data ( which remains optional ), to flip the attributes intelligently, or in other words, according to a policy, to avoid the exhaustive search.
We begin by explaining what is Q-Learning:
Q-learning is a model-free, off-policy reinforcement learning algorithm proposed by (Watkins & Dayan, 1992). It consists of evaluating the goodness of a state-action pair. Q-value at state s and action a, is defined the expected cumulative reward from taking action a in state s and then following the policy.
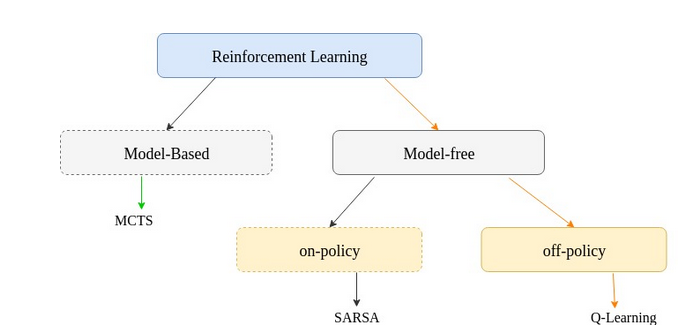
Q-Table
Q-Table is just a fancy name for a simple track-keeping table, like ADL said in his article. It consists generally of a 2D-array, where the rows represent the states and the columns, the possible actions, and because when you deal with feature selection, it’s not wise to use a static data structure, so I am using a list[the index is nbrOnes(state)] of dictionaries[the key is “state”] of dictionaries[the key is “action”] ( a dictionary or dict in python, is like a HashMap in JAVA )
q_table[nbrOnes(state)][“state”][“action”]
Q-function
The Q-learning algorithm is based on the Bellman Optimality Equation given below :
Q(state, action) = R(state, action) + Gamma * Max[Q(next state, all actions)]
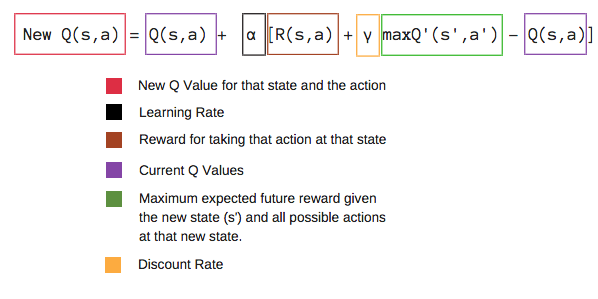
And that’s how we update the table each time, and we use the q-values to pick the next state, which is in this case, what attributes to flip, and here’s the Q-LocalSearch function :
def ql_localSearch(self):for itr in range(self.locIterations):state = self.solutionself.fitness = self.data.evaluate(state)if not self.data.ql.str_sol(state) in self.data.ql.q_table[self.data.ql.nbrUn(state)]:self.data.ql.q_table[self.data.ql.nbrUn(state)][self.data.ql.str_sol(state)] = {self.data.ql.str_sol(state):{}}action = self.data.ql.step(self.data,state)next_state = self.data.ql.get_next_state(state,action)accur_ns = self.data.evaluate(next_state)self.data.ql.learn(self.data,state,action,self.fitness,next_state)self.solution = next_state.copy()
Recap
To recap what we’ve done here today, we talked about Q-learning, and how we used it to make a so called Q-LocalSearch function, which is a way to use Q-learning algorithm for feature selection.
And for this model we have :
- state : is a combinaison of features
- action : flipping a feature
- reward : for now, we consider the accuracy ( fitness ) of the state-action as a reward, but in the next article maybe, we will go deeper and see why it’s not the optimal way to define it.
I really hope you enjoyed it, I’m trying to put everything that I judge important, and if you need more details do not hesitate to leave a comment, or PM me.
And yeah, you can always check my last article.